Profit scoring for credit unions using the multilayer perceptron, XGBoost and TabNet algorithms: evidence from Peru
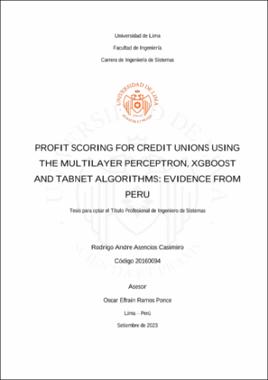
Ver/
Tesis_resumen
(application/pdf: 223.5Kb)
(application/pdf: 223.5Kb)
Tesis
(application/pdf: 273.8Kb)
(application/pdf: 273.8Kb)
Autorización
(application/pdf: 205.1Kb)
(application/pdf: 205.1Kb)
Reporte de similitud
(application/pdf: 1.578Mb)
(application/pdf: 1.578Mb)
Fecha
2023Autor(es)
Asesor(es)
Metadatos
Mostrar el registro completo del ítemResumen
Las Cooperativas de Ahorro y Crédito son instituciones microfinancieras en crecimiento que basan sus decisiones de préstamo en el juicio de sus analistas de crédito. Por ello, el objetivo de este trabajo es diseñar 6 modelos de profit scoring, capaces de predecir la Tasa Interna de Retorno (TIR) de las solicitudes de crédito, utilizando los algoritmos del perceptrón multicapa, XGBoost y TabNet, y servir, así, como herramienta de soporte al analista de crédito. Para ello, se seleccionaron las características menos correlacionadas y más independientes del conjunto de datos proveniente de una Cooperativa de Ahorro y Crédito peruana y compuesto por 36 402 observaciones. Luego, se optimizaron los hiperparámetros de todos los algoritmos. Finalmente, se compararon los modelos de profit scoring que consideraron solo las características seleccionadas con los que consideraron todas las características. Como resultados, se obtuvo que las características más significativas que determinan la TIR de un préstamo son la tasa efectiva mensual y los días de morosidad máxima o media del socio. Los resultados obtenidos de la evaluación del rendimiento de los modelos de profit scoring sugirieron a XGBoost como el mejor algoritmo. Además, el modelo que utilizó el algoritmo XGBoost y que consideró todas las características obtuvo el mejor rendimiento. Credit unions are growing microfinance institutions that base their lending decisions on the judgment of
their credit analysts. Therefore, the purpose of this paper is to design 6 profit scoring models, capable of predicting the Internal Rate of Return (IRR) of credit applications, using the multilayer perceptron, XGBoost and TabNet algorithms and thus serve as a support tool for the credit analyst. For this purpose, the least correlated and most
independent features were selected from the dataset coming from a Peruvian credit union and composed of 36 402
observations. Then, the hyperparameters of all algorithms were tuned. Finally, the profit scoring models that considered only the selected features were compared to which considered all features. As results, it was obtained that the most significant features that determine the IRR of a loan are the effective monthly interest rate and the member’s maximum or average days delinquent. The results obtained from the performance evaluation of the profit scoring models suggested the XGBoost as the best algorithm. In addition, the model that used the XGBoost algorithm and considered all the features had the best performance.
Cómo citar
Asencios Casimiro, R. A. (2023). Profit scoring for credit unions using the multilayer perceptron, XGBoost and TabNet algorithms: evidence from Peru. [Tesis para optar el Título Profesional de Ingeniero de Sistemas, Universidad de Lima]. Repositorio institucional de la Universidad de Lima. https://hdl.handle.net/20.500.12724/19751Editor
Universidad de LimaNota
El acceso al texto completo de la tesis estará habilitado el 23 de noviembre de 2025, por expresa disposición del autor.
Coleccion(es)
- Tesis [43]