Semantic Segmentation of Weeds and Crops in Multispectral Images by Using a Convolutional Neural Networks Based on U-Net
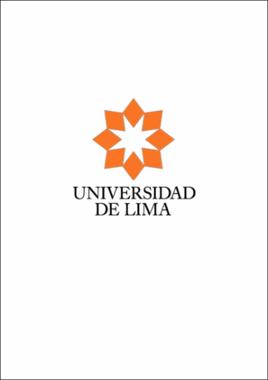
Ver/
Logo_ULima.pdf
(application/pdf: 49.99Kb)
(application/pdf: 49.99Kb)
Fecha
2020Metadatos
Mostrar el registro completo del ítemResumen
A first step in the process of automating weed removal in precision agriculture is the semantic segmentation of crops, weeds and soil. Deep learning techniques based on convolutional neural networks are successfully applied today and one of the most popular network architectures in semantic segmentation problems is U-Net. In this article, the variants in the U-Net architecture were evaluated based on the aggregation of residual and recurring blocks to improve their performance. For training and testing, a set of data available on the Internet was used, consisting of 60 multispectral images with unbalanced pixels, so techniques were applied to increase and balance the data. Experimental results show a slight increase in quality metrics compared to the classic U-Net architecture.
Cómo citar
Chicchon Azapa, M. & Bedón Monzón, H. (2020). Semantic Segmentation of Weeds and Crops in Multispectral Images by Using a Convolutional Neural Networks Based on U-Net. Communications in Computer and Information Science. 473-485. https://link.springer.com/chapter/10.1007%2F978-3-030-42520-3_38Editor
SpringerÁrea / Línea de investigación
Productividad y empleo / Innovación: tecnologías y productosTemas
Nota
Indexado en Scopus
Coleccion(es)
- Ingeniería Industrial [204]